- Fintech Brainfood
- Posts
- 🧠Moody's the 115 Year Old Growth Fintech Company
🧠Moody's the 115 Year Old Growth Fintech Company
Plus; Klarna's path to profit helped by AI, Nubank keeps crushing and don't look now but Bitcoin is approaching it's all time high.
Welcome to Fintech Brainfood, the weekly deep dive into Fintech news, events, and analysis. Join the 38,564 others by clicking below.
Hey Fintech Nerds 👋
What a week!
The good, Klarna is getting more profitable, Nubank is crushing and even Marqeta is doing well.
The bad was more enforcement action against banks, exiting relationships between BaaS providers, and sadly, Treasury Prime let go of half of its staff.
We’re still in the messy middle of Fintech’s renaissance.
But the Fintech that does make it, will put a dent in the industry.
NuBank’s $1bn profit is a warning shot for the banking industry.
They’re on track to be the most profitable bank in Brazil in 2025 with over 100m consumers, and they’ve barely started to cross-sell. My take? This trend will come to all digital banks over the next decade. Watch what Square does with CashApp.
Klarna’s AI does the work of 700 agents, resolves customer problems in 2 minutes instead of 11, is 24/7, and speaks 35 languages. I give it a year until this is normal in Fintech and 5 years until banks do it.
Oh, and don’t look now, but Bitcoin is skyrocketing again.
I have opinions as always (in things to know).
This week’s Rant was super fun too. Moody’s is the Fintech Growth story nobody talks about and it is fascinating.
PS. Shout out to the incredible Jason Mikula for being first with everything happening in embedded finance. Man is a machine.
Here's this week's Brainfood in summary
📣 Rant: Moody's the 115 Year Old Growth Fintech Company
💸 4 Fintech Companies:
👀 Things to Know:
📚 Good Read: Building a ledger system
Weekly Rant 📣
Moody's the 115 Year Old Growth Fintech Company
Looking at the top 10 Fintech Growth stocks, 4 out of 10 are in financial markets data or analytics.

That one just squeezing into the top 10 is a 115-year-old company. Moody's.
Like anything that lasts more than one market cycle, there are lessons to take. The consistently high-performing financial stocks league table has multiple companies that combine data + risk + credit.
The last decade of Fintech was mostly payments.
The next decade will be about data and risk.
All risk problems are data science problems.
Moody's has built a moat around data to price corporate and government debt.
Making it the most interesting Fintech company hidden in plain sight.
Why Moody's is a Growth Company
Rule of 40, revenue growth, retention
Moody's Origin Story
Founded in 1909
Early railroad ratings
Regulatory boost in 1930s
Dominance by 1970s
The Ratings Business
Ratings are cyclical
Birth of Analytics business
Analytics delivers recurring revenue
Growth through acquisitions
Moody's Data Moat
Started with better railroad data
Now has 100+ years of global data
Creates network effects and switching costs
Lessons for Fintech
Need better risk data
Build proprietary data assets
Create "rating" systems
Watch for disruption of incumbents
Who Could Be Next?
Business lending data
Digital KYB/AML/fraud data
Key is proprietary data + risk models
1. Why is Moody's a growth company?
The Rule of 40 says revenue growth + EBITA should equal or exceed 40. Moody's is growing revenue by ~10 % YoY with a consistent 35%+ EBITDA. ✅
Net Revenue retention isn't bad either. Not quite enterprise SaaS, but they're in the right ballpark. Moody's has a 90% Net Revenue Retention Rate (NRR). ✅
Moody's is one of the big 3 corporate and sovereign rating agencies. Instead of giving a consumer a credit score of "675" like FICO or VantageScore would. Moody's provides a score like Aaa or Aa1 to Coca-Cola or Pepsico for a specific bond (debt) issuance.

Alongside Standard and Poors (S&P) and Fitch, the big three dominate the US and international debt ratings market. This market is highly cyclical; when there are many borrowers, times are good, and fewer borrowers are bad.
Moody's stands out because it doesn't just do ratings. Its analytics business has become an engine of growth and subscription revenue. Taking the data it had about credit, it could repackage this as software and sell analytics about credit and compliance risk to lenders and financial institutions.
2. Moody's 1907 to today.
John Moody began publishing analyses of stocks and bonds and established the self-titled John Moody Company. It detailed the stocks and bonds owned by governments, businesses, and banks before being forced to sell in the 1907 financial crisis.
The 1907 crisis was linked to a run on banks that had lent heavily to the often with little or no underwriting and the creation of the Federal Reserve. The easy pre-crisis lending created a boom in railroad bonds, but investors could not understand the quality of those loans as an investment.
Moody's as we know it today was founded in 1909 with a publication titled Analysis of Railroad Investments. Investors' thirst for data they could rely on was at a peak. Rating that debt gave Moody's an edge.
💡 Insight #1: High-quality credit rating data is critical for markets to function
Following the great depression, the Glass-Stegall app created the FDIC to insure depositors and separated commercial from investment banks. Any bank overseen by the Federal Reserve could only hold investment-grade securities.
An investment-grade security (stock or bond) is one rated by Fitch, Moody's, or S&P. For Moody's, an investment grade security is between Aaa and Baa3.
Side note: Dun & Bradstreet acquired Moody's in 1962. However, the two companies operated independently, leading to Moody's eventual spin-out and listing in 1999.
💡 Insight #2: Banks now needed the rating to know what they could hold to comply with legislation.
1975 to 2007.
From 1967 to 1968, more than 160 companies failed due to inadequate risk management. So, In 1975, the SEC introduced new capital requirements for broker-dealers (companies who sell securities like bonds but also trade them).
These rules required, at a minimum, an "A" rating from a nationally recognized statistical ratings organization (NRSRO), which was initially a list of 3. That list later grew, but by that point, the "big 3" had a dominant market position.
💡 Insight #3: The rating was now required by regulation.
Moody's 2007 to today.
A lot happened in 2007.
Lehman Brothers had been rated "A" by all three rating agencies.
Collateralized Debt Obligations (CDOs) would often carry "Aaa" or equivalent ratings despite containing risky loans among 100s or 1000s mortgages. Moody's downgraded 83% of products that had received Aaa ratings in 2006.
After the Great Financial Crisis, regulators tried breaking up the three rating agencies. The Dodd-Frank Act of 2010 increased oversight of these agencies, but their market dominance hasn't budged since then.
💡 Insight #4: The network effect and scale is too great even for regulation to constrain
So let's recap
Moody's was one of three companies required by regulation to rate corporate and sovereign debt.
Moody's had over 100 years of proprietary data credit history.
Moody's ratings had become one of three technical languages known and used by professional investors.
All fixed income is measured with this language. Moody's has a grip on how investors think, as well as a moat of both data and regulation.
Moody's data shows how many mid-sized corporations refinanced or defaulted on bonds the last time rates went up 5% in 18 months.
💡 Insight #5: With over 100 years of history, Moody's data shows how debt performs in a variety of market conditions.
3. The problem with the rating business is that it is cyclical.
Lots of new debt or re-rating creates a good year; a lack of it creates a bad year. Moody's needed something evergreen and recurring.
The solution was to create a new business unit called Moody's Analytics.
The new unit rolled together software Moody's sold to clients to help its clients accurately asses and price credit risk with other acquisitions. Moody's Analytics has delivered 16 years of QoQ revenue growth.
Driven in part by acquisitions that increased dramatically from 2017.
From 2010 to 2020, Moody's acquired 12 companies mostly focussed on KYC and compliance, acquiring RDC, Kompany, Passfort, and Bureau van Dijk. KYC and AML products are now the fastest-growing segment of the entire business.
💡 Insight #6: Moody's used M&A to get more data and drive more value from its existing data
4. The Moody's MOAT
Today, all fixed-income financial products are priced using the rating language of Fitch, Moody's, or S&P. Moody's consistently leverages its data moat and enterprise relationships to solve new problems with data.
Moody's has
High switching costs: Changing the rating provider would mean taking a risk on a rating that has less data to rate from.
Network effects: Most investors, regulators, and debt issuers know of and work with the rating agencies and understand what a rating means.
Regulatory advantage: While regulators recognize other rating agencies, the big three gained huge traction from being recognized as NRSROs.
Efficient scale: The data from over 100 years of rating creates a quality and trust that is hard to dislodge. It also gives them a position with most of the big lenders in the world's largest capital market (the USA).
Data about risk: This isn't a business school moat, but it's fair to say Google and Meta have proven data moats can be valuable. Moody's has a similar moat around its data.
This last point is the killer insight.
💡 Insight #7: Risk data is the most valuable asset in Fintech because everything in finance is risk.
Every transaction in finance has some element of risk. A payment could be an error or fraud, lenders might not get repaid, and investors might lose value instead of growing their investments. Each data fragment is a tiny puzzle piece helping you build a more accurate picture of risk. With data about the risk, you can lend more profitably, reduce losses, and prevent fraud more effectively.
Pricing risk isn't just about having a lot of data; it's about having high-value and high-quality data.
💡 Insight #8: All risk problems are data science problems. Most data science problems can be solved with higher quality if you get better data and more data.
Take consumer credit as an example.
Traditional lenders have much more data than new market entrants. They've lent to consumers over decades and know how to price risk in good and bad markets. Modern lenders might use better data, such as the cash flow from open banking or payroll data from companies like Pinwheel or Atomic.
Moody's is an example of a company that started with better data about railroad companies when the market needed it. Over time, it has evolved to have much more data with over 100 years of an elusive data set. How Governments, financial institutions, and corporations perform across good and bad markets.
Through acquisition, it has added demographics, ESG, political, company information, and regulatory data with a global perspective. It is constantly hunting for better data and more data across the risk spectrum.
Maybe one day, all B2B Fintech risk companies get big enough to be acquired by Moody's?
Can anyone (other than Fitch and S&P) compete?
5. Who's building the next Moody's?
The past decade saw new payment companies like Stripe take market share from incumbent competitors.
Similarly, we could see new players in business credit risk data follow Moody's playbook to disrupt incumbent providers like Moody's itself. This might take decades to play out, but the idea fascinates me.
New companies could gather proprietary data in emerging areas, like B2B fintech lending. Players like Brex and Ramp have lent billions of dollars using non-traditional data sources. Will someone bundle these new insights into a 'B2B Fintech Risk Rating' system and take market share?
And just as Moody's ratings became a universal language, might we see new standards emerge from these disruptors? Imagine an 'AAA' for B2B fintech borrowers with proven recurring revenue streams. Or a 'BBB' for early-stage SaaS companies with fluctuating cash flow.
Moody's path suggests the winners will first amass proprietary data on new sectors, then develop the dominant risk rating language.
But even if they did succeed in an initial wedge, you can bet Moody's will come along and try to acquire.
We're still so early in B2B Risk data more broadly.
Digital KYB is super early.
Middesk and its competitors are doing a great job pulling together disparate data sets about companies, but they're very US-focused. It wouldn't work for a global marketplace with an HQ in the US. Nor is it easily repackaged for global onboarding. (Note also, Moody’s acquired Kompany, which operates in this space).
Digital fraud prevention, transaction monitoring, and scam detection are all in their infancy for most B2B transaction types because, until recently, they were manual and slow. As the world goes digital, so does risk.
Many techniques pioneered for consumer Fintech will come to B2B, but it will take time.
The lesson from Moody's are
Be intentional about building a data moat. Your wedge is better data; your goal is more data.
Don't be shy about M&A. There aren't many Fintech companies with the capital stack to go on a buying spree, but as the IPO window opens, that could change.
Develop a language that becomes industry standard. What's the language for an embedded SaaS lending program that's great at lending? A***?
The challenge.
So, who's collecting the best data?
And what problem are they solving?
Because there lays a clue to what comes next.
ST.
4 Fintech Companies 💸
1. Cambio - The AI Agent that improves your credit
Cambio is an AI-powered chatbot that negotiates with lenders to help consumers improve their credit score and financial position. For $8.33 per month (or $99.66 per year), and signed power of attorney the app will call collectors on behalf of users and negotiate a debt. The company has also started to work with banks and credit unions to handle customer comms as a chatbot via multiple channels.
🧠People hate negotiating with lenders. Being in debt is stressful, and being behind on payments is even worse. This is an ideal use case for a consumer-advocacy focussed chatbot. The real opportunity for Cambio will be in customer outreach on behalf of banks. A consumer app can only go so far. Collections are a huge brand opportunity for banks, if you do that thoughtfully and well, it's also a great way to build the relationship. Then later down the line begin to cross-sell. Robo calling for junk advertising has been declared illegal by the FCC, but if its genuine consumer advocacy, will we get a different outcome?
2. Provisian - Financial planning dashboard powered by open banking
Provisian aggregates checking, lending, and investment accounts into a wealth advisor grade dashboard. Users can model scenarios like consolidating loans or what changing pension contributions looks like over time. It covers income tax filling projections and current and future expenses and outputs a "net worth report." The product is aimed at Registered Investment Advisors and prosumers.
🧠Provisian looks at Mint.com, and wealth managers like Schwaab took their spreadsheets and had a baby. It is a complete dashboard of all assets, liabilities, and scenarios. This makes those bar charts on the homepage of a Robo-advisor look redundant. I kinda want this but with way more automation. I hope this grade of insight becomes the norm
3. Kite - Founder-friendly capital for MENA
Kite provides lending to SME marketplaces, e-commerce stores, retailers, and subscription billers. Using data as input, they can reduce the operational cost of lending, which brings these smaller businesses into profitability.
🧠Underwriting in high-risk markets is non-trivial. It looks like data-driven financing or revenue-based finance, which is much more common in the world of Fintech now. From multiple data points, core bits of Underwriting can be automated. Of course, the danger with any new lending business is lending is easy, getting paid back is hard, and having a supply of capital to lend is harder. This team has a strong lending background, so I'm excited to see what they can do.
4. Revenew - The Payments Ops platform for marketplaces going multi-PSP.
Revenue is the payment ops platform that helps marketplaces and platforms easily manage multiple payment service providers (PSPs). It helps manage complex payment flows, automates reconciliation, and provides a clear dashboard of unit economics. Users can also create a consistent onboarding experience and have fine-grained pricing controls.
🧠There comes a time in every company that does payments life when it scales to needing multiple PSPs and to bring its ledger in-house. It could be for market expansion, cost, reconciliation, or just to avoid being locked in with a single PSP. Many larger platforms and marketplaces built this themselves. Even with the glut of payment automation and workflow tools, there was still a decision-making and dashboard gap.
Things to know 👀
Nubank posted a full year of $1bn profit on $8bn revenue. The bank added 20m customers in the 12 months with a return on equity (RoE) of 23%. The bank is now focused on moving up the market and increasing its average revenue per user (ARPU) from $26. It's on track to hit a RoE of 30% in 2024, making it the most profitable bank in LATAM.
🧠This kills the big argument that "disruptors aren't profitable." Digital banks are more profitable than big banks. For context, the largest bank in Brazil by assets, Itau Unibanco, has an RoE of 21%. JP Morgan delivered around 10 to 15% across 2023. Outside the big names in Europe and the US, a RoE of 6 to 10% is normal.
🧠It's a unit economics thing. The cost to acquire and serve a Nubank customer is a fraction of any other bank. It costs Nubank $5 to acquire a customer, and their cost to serve is less than $11 annually.
🧠Their revenue potential will increase from here. They've also developed much more cross-selling into lending products, which means they can increase the revenue per user.
🧠Expect this trend to repeat globally. Consumer digital banks and Neobanks have similar low-cost bases in the US and Europe and are much earlier in the cycle, turning on new revenue lines. Now Jack Dorsey is back in charge at Square; it's something he's aggressively pushing at Square, too.
🧠It annoys me how often I hear, "oh well, the big banks will do this better." Most banks are in denial publicly but scrambling internally to figure out how to copy Nubank's success. The reality is they can't. Not with the systems, processes, and culture they have today. Growth is a willingness to change and the ability to change.
2. Klarna is displacing 700 jobs with AI. / Path to profitability.
The new assistant had 2.3m conversations and handled two-thirds of Klarna's customer conversations. It is doing the work of 700 full-time agents more accurately (with a 25% drop in repeat inquiries) and solving customer problems in 2 minutes instead of 11. It is 24/7 and speaks 35 languages, which is estimated to save Klarna $40m.
It also announced revenue up 22%, credit losses down 32%, and a net loss of $241m, down from $1bn the year earlier.
🧠This proves that AI takes jobs and performs them better than humans. Klarna's CEO noted we need to start a social conversation about handling the coming impact.
🧠Chatbots used to suck. It became frustrating whenever customer service websites tried to triage with a bot. The key with LLM-powered bots is in the fine-tuning for the use case. Vanilla ChatGPT results may vary, but it is very effective for cost-saving in this basic use case.
🧠We're seeing job losses in the tech sector first because they're early adopters. Banks and traditional industries today rely on outsourced and offshore talent. The incumbents being disrupted here are the major outsourcers like Wipro, Capgemini, and Genpact.
🧠I give it ~1 year until a bank does this.
🧠Like dot com, AI is a short-term bubble. Like the dot com, it will reshape society, geopolitics, and the economy in the long term. This is why talk of "regulation" isn't stupid. Governments have learned social media lessons and are keen to get ahead of that type of issue. But to the Klarna CEO's point. We're not having the conversation at a high enough level about what the world looks like in 10 years.
Bitcoin passed $63,000 on Thursday following multiple 10% jumps in 24 hours. This follows the recent launch of ETF funds from companies like Blackrock and Fidelity.
🧠Bitcoin is quietly joining the ranks of legitimate assets. The amount of haters is dwindling. Fidelity now allocates 1% of its "conservative" fund in Canada to Bitcoin. If that rolls out globally, this is just the beginning.

🧠I really hope we learn the lessons of previous Crypto cycles. It's time to end the "love/hate" Crypto conversation and accept it as a reality. Now it's a question of how to ensure it's well risk managed and minimize consumer harm.
Good Reads 📚
Stripe's ledger service is a beast. On Black Friday weekend, it processed 300m transactions with $18.6Bn of volume. The problem is the outside world is inconsistent; banks and other players send errors and malformed data. Stripe automatically verifies 99.99% (4 nines) of all transactions and has built tooling that manages the long tail (up to 99.999%). Stripe built two things.
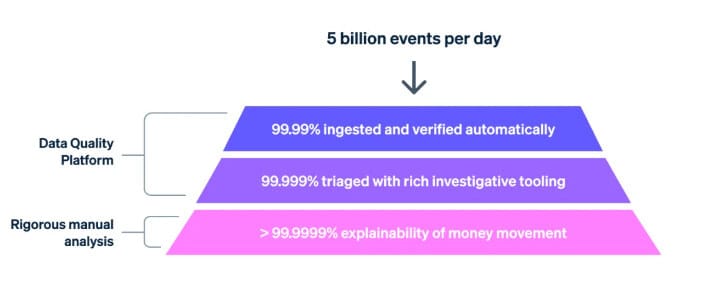
A state machine is representing external systems (like payments rails, a merchant, or banks) and their fund flows.
A computed estimation of balances to evaluate the health of the external system
🧠Stripe has modeled the reliability of the external world as software. If this ran at the core of every bank, we'd likely add 1% to GDP in efficiency gains alone. So much of the time and energy in banks is spent fixing weird reconciliation errors and missing matches against the outside world.
🧠Why should you read this? Building a ledger is hard. Everyone starts out thinking, "Oh, it's an event log; that's easy." Wrong. The world is complex, and the waterfalls you have to build around that to hit scale are substantial.
🧠If you're in finance, you'll eventually need a ledger. You choose to build one like Stripe has or use a tool like Fragment.dev to do it.
🧠My big takeaway is how to model accounting and payments as data. That is an art form that is increasingly becoming an engineering science.
That's all, folks. 👋
Remember, if you're enjoying this content, please do tell all your fintech friends to check it out and hit the subscribe button :)
(1) All content and views expressed here are the authors' personal opinions and do not reflect the views of any of their employers or employees.
(2) All companies or assets mentioned by the author in which the author has a personal and/or financial interest are denoted with a *. None of the above constitutes investment advice, and you should seek independent advice before making any investment decisions.
(3) Any companies mentioned are top of mind and used for illustrative purposes only.
(4) A team of researchers has not rigorously fact-checked this. Please don't take it as gospel—strong opinions weakly held
(5) Citations may be missing, and I've done my best to cite, but I will always aim to update and correct the live version where possible. If I cited you and got the referencing wrong, please reach out